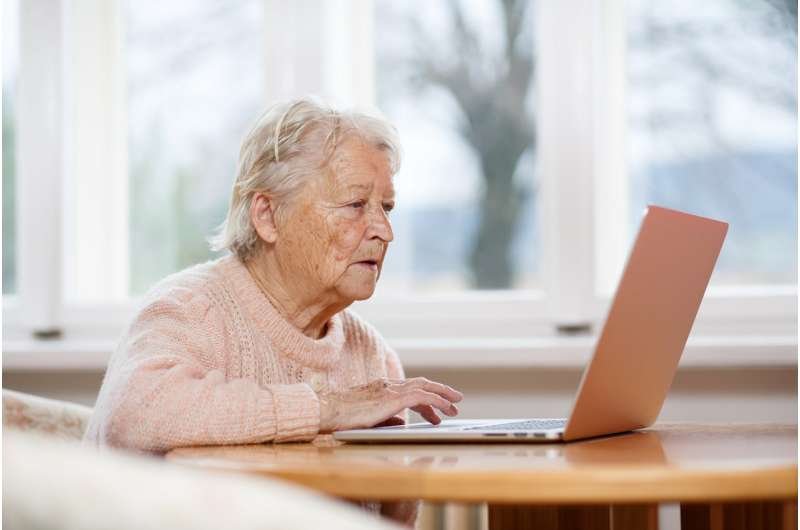
Investigating the Readability, Linguistic Psychological and Emotional Characteristics of Digital Dementia Information
This study investigates the readability, linguistic characteristics, emotional tone, and target audiences of English-language digital dementia information. Researchers analyzed 300 medical articles, 35 websites, and 50 blogs using readability metrics (Flesch Reading Ease and Flesch-Kincaid Grade Level), linguistic analysis (LIWC), and thematic categorization.
A thematic analysis was conducted to categorize target audiences. Results showed that digital dementia information had a high median readability score (Grade Level 12.1), making it difficult for the general public to comprehend. Medical articles were the most analytical but had lower authenticity and emotional tone compared to websites and blogs.
Blogs exhibited higher authenticity but lower analytical scores than websites. Most blogs and webpages were targeted at people with dementia, but content aimed at general audiences had the lowest readability, making it less accessible. Website information for caregivers had higher authority (clout) but lower authenticity.
The study concludes that while there is ample dementia-related content, it is largely inaccessible to those with lower education levels, which is concerning given their increased dementia risk. Additionally, the predominantly negative tone of the content may contribute to emotional distress among those diagnosed.
Emotion analysis in LIWC identifies and quantifies emotional expressions in text by categorizing words into positive and negative emotion categories. It helps researchers analyze sentiment, mood, and psychological states by detecting emotional tone variations in written or spoken language. This feature is widely used in psychology, social sciences, and computational linguistics to study emotional patterns in communication.
Text’s Emotion Analysis: LIWC
Linguistic Inquiry and Word Count (LIWC) is a text analysis tool designed to examine word usage and gain insights into psychological, emotional, and social aspects of communication. It categorizes words into linguistic, psychological, and topical dimensions, helping researchers understand thought patterns, personality traits, and sentiment. LIWC is widely used in psychology, linguistics, and social sciences for analyzing written and spoken language. The latest version, LIWC-22, offers advanced features such as real-time analysis, visualization tools, and integration with Python and R. With over 20,000 scientific studies utilizing LIWC, it remains a trusted tool for text-based research.